Os dados¶
Para coletar os dados de outros países, foram utilizadas as APIs dos sites:
[1]:
import requests
import pandas as pd
covid_api = 'https://corona-api.com/countries/'
rest_countries = 'https://restcountries.eu/rest/v2/alpha/'
country = 'CN' # Alpha-2 ISO3166
data_json = requests.get(covid_api + country).json()
country = requests.get(covid_api + country).json()
N = country['data']['population']
print(country['data']['name'])
China
Organizando os dados¶
[2]:
from datetime import datetime
df = pd.DataFrame(data_json['data']['timeline'])
df = df.sort_values('date').reset_index()
from datetime import datetime, timedelta
df['date'] = [datetime.fromisoformat(f) for f in df['date']]
df = df.drop_duplicates(subset='date', keep = 'last')
# Criando o vetor de tempo
first_date = df['date'].iloc[0]
size_days = (df['date'].iloc[-1] - df['date'].iloc[0]).days
date_vec = [first_date + timedelta(days=k) for k in range(size_days)]
new_df = pd.DataFrame(date_vec, columns=['date'])
new_df = pd.merge(new_df, df, how='left', on= 'date')
new_df = new_df.drop(columns= ['index', 'updated_at', 'is_in_progress'])
for col in new_df.columns[1:]:
new_df[col] = new_df[col].interpolate(method='polynomial', order=1)
df = new_df.dropna()
df.head()
[2]:
date | deaths | confirmed | active | recovered | new_confirmed | new_recovered | new_deaths | |
---|---|---|---|---|---|---|---|---|
0 | 2020-01-21 | 17 | 548 | 503 | 28 | 548 | 28 | 17 |
1 | 2020-01-22 | 18 | 643 | 595 | 30 | 95 | 2 | 1 |
2 | 2020-01-23 | 26 | 920 | 858 | 36 | 277 | 6 | 8 |
3 | 2020-01-24 | 42 | 1406 | 1325 | 39 | 486 | 3 | 16 |
4 | 2020-01-25 | 56 | 2075 | 1970 | 49 | 669 | 10 | 14 |
Visualizando os dados¶
[3]:
from bokeh.models import Legend, ColumnDataSource, RangeTool, LinearAxis, Range1d, HoverTool
from bokeh.palettes import brewer, Inferno256
from bokeh.plotting import figure, show
from bokeh.layouts import column
from bokeh.io import output_notebook
output_notebook()
import numpy as np
# Criando os valores para legenda no plot
year = [str(int(d.year)) for d in df['date'] ]
month = [("0"+str(int(d.month)))[-2:] for d in df['date'] ]
day = [("0"+str(int(d.day)))[-2:] for d in df['date'] ]
# Criando a fonte de dados
source = ColumnDataSource(data={
'Data' : df['date'].values,
'd': day, 'm': month, 'y': year,
'Infectados Acc' : df['confirmed'].values,
'Mortes' : df['deaths'].values,
'Ativo' : df['active'].values,
'Recuperados': df['recovered'].values
})
# Criando a figura
p = figure(plot_height=500,
plot_width=600,
x_axis_type="datetime",
tools="",
#y_axis_type="log",
toolbar_location=None,
title="Evolução do COVID - " + country['data']['name'])
# Preparando o estilo
p.grid.grid_line_alpha = 0
p.ygrid.band_fill_color = "olive"
p.ygrid.band_fill_alpha = 0.1
p.yaxis.axis_label = "Indivíduos"
p.xaxis.axis_label = "Dias"
# Incluindo as curvas
i_p = p.line(x='Data', y='Ativo',
legend_label="Infectados",
line_cap="round", line_width=5, color="#c62828", source=source)
m_p = p.line(x='Data', y='Mortes',
legend_label="Mortes",
line_cap="round", line_width=5, color="#512da8", source=source)
c_p = p.line(x='Data', y='Infectados Acc',
legend_label="Infectados Acc",
line_cap="round", line_width=5, color="#0288d1", source=source)
r_p = p.line(x='Data', y='Recuperados',
legend_label="Recuperados",
line_cap="round", line_width=5, color="#388e3c", source=source)
# Colocando as legendas
p.legend.click_policy="hide"
# p.legend.location = "top_left"
p.legend.location = "top_left"
# Incluindo a ferramenta de hover
p.add_tools(HoverTool(
tooltips=[
( 'Indivíduos', '$y{i}'),
( 'Data', '@d/@m/@y' ),
],
renderers=[
m_p, i_p, c_p, r_p
]
))
show(p)
Verificando os dados¶
[4]:
dif_I = np.diff(df['active'])
cum = []
cum.append(dif_I[0])
for k, i in enumerate(dif_I):
cum.append(cum[-1] + i)
cum = np.array(cum)
cum += df['deaths'].to_numpy() + df['recovered'].to_numpy()
print("Erro entre casos acumulados e valores de confirmados: {}".format(
round(sum((cum - df['confirmed'].values)**2 / len(cum)),2) ) )
Erro entre casos acumulados e valores de confirmados: 168921.0
Criando os dados SIR¶
[5]:
I = df['active'].to_numpy()
R = df['recovered'].to_numpy()
M = df['deaths'].to_numpy()
S = N - R - I
# Creating the time vector
t = np.linspace(0, len(I), len(I))
Sd, Id, Md, Rd, td = S, I, M, R, t
[7]:
Ro = (np.log(S[0]/N) - np.log(S[-1]/N))/(S[0]/N - S[-1]/N)
print("Calculo do Ro baseado nos dados:", Ro)
Calculo do Ro baseado nos dados: 1.0000300994523579
Estimando utilizando todos os dados¶
[8]:
from models import *
dataset = dict(S=Sd, I=Id, R=Rd)
# Create the model
sir_model = ss.SIR(pop=N, focus=["S", "I", "R"])
# Adjust the parameters
sir_model.fit(dataset, td,
search_pop=True,
pop_sens=[0.00001,0.05],
beta_sens=[100,10000],
r_sens=[100,100])
# Predict the model
sim_res = sir_model.predict((Sd[0],Id[0],Rd[0]), td)
├─ S(0) ─ I(0) ─ R(0) ─ [1330043469, 503, 28]
├─ beta ─ 1 r ─ 0.14285714285714285
├─ beta bound ─ 0.01 ─ 10000
├─ r bound ─ 0.0014285714285714286 ─ 14.285714285714285
├─ equation weights ─ [7.51893117169047e-10, 7.90340642628301e-05, 1.8154828650455473e-05]
├─ Running on ─ differential_evolution SciPy Search Algorithm
└─ Defined at: 5100.406034312756 ─ 0.04454555270373974
[9]:
print("Parâmetros estimados: ", sir_model.parameters)
print("Suposto Ro: ", sir_model.parameters[0] * sir_model.parameters[-1] / sir_model.parameters[1])
print("Dias contaminados: ", 1 / sir_model.parameters[1])
Parâmetros estimados: [5.10040603e+03 4.45455527e-02 6.14351607e-05]
Suposto Ro: 7.034243490574524
Dias contaminados: 22.448930124421754
[14]:
p = figure(plot_height=500,
plot_width=800,
tools="",
toolbar_location=None,
title="Evolução do COVID - " + country['data']['name'])
# Preparando o estilo
p.grid.grid_line_alpha = 0
p.ygrid.band_fill_color = "olive"
p.ygrid.band_fill_alpha = 0.1
p.yaxis.axis_label = "Indivíduos"
p.xaxis.axis_label = "Dias"
p.line(t, I,
legend_label="Infectados", color="#ff6659", line_width=4)
p.line(t, R,
legend_label="Removidos", color="#76d275", line_width=4)
# Show the results
p.line(td, sim_res[1],
legend_label="Infectados - Modelo", line_dash="dashed", color="#d32f2f", line_width=3)
p.line(td, sim_res[2],
legend_label="Removidos - Modelo", line_dash="dashed", color="#43a047", line_width=3)
p.line(td, sim_res[0],
legend_label="Suscetíveis Ponderados - Modelo", line_dash="dashed", color="#1e88e5", line_width=3)
p.add_layout(p.legend[0], 'right')
show(p)
Monte Carlo¶
Nesta parte, faremos um teste aumentando a quantidade de amostras de treinamento e prevendo o momento do pico da epidemia a medida que mais dias são utilizados para treinamento. Esse estudo vai possibilitar a análise da certeza da previsão do pico da epidemia antes desse acontecer.
[15]:
saved_param = {'r':[], 'beta':[], 'pop':[]}
saved_prediction = []
start_day = 10
pred_t = np.array(range(int(td[-1])))
for i in range(start_day, len(I)):
dataset = dict(S=Sd[:i], I=Id[:i], R=Rd[:i])
td_ = td[:i]
# Create the model
sir_model = ss.SIR(pop=N, focus=["S", "I", "R"], verbose = False)
# Adjust the parameters
sir_model.fit(dataset, td_,
search_pop=True,
pop_sens=[0.00001,0.05],
beta_sens=[100,10000],
r_sens=[100,100])
saved_param['beta'].append(sir_model.parameters[0])
saved_param['r'].append(sir_model.parameters[1])
saved_param['pop'].append(sir_model.parameters[2])
saved_prediction.append(sir_model.predict((Sd[0],Id[0], Rd[0]), pred_t))
/opt/anaconda3/lib/python3.7/site-packages/scipy/integrate/odepack.py:248: ODEintWarning: Excess work done on this call (perhaps wrong Dfun type). Run with full_output = 1 to get quantitative information.
warnings.warn(warning_msg, ODEintWarning)
/opt/anaconda3/lib/python3.7/site-packages/scipy/integrate/odepack.py:248: ODEintWarning: Excess work done on this call (perhaps wrong Dfun type). Run with full_output = 1 to get quantitative information.
warnings.warn(warning_msg, ODEintWarning)
/opt/anaconda3/lib/python3.7/site-packages/scipy/integrate/odepack.py:248: ODEintWarning: Excess work done on this call (perhaps wrong Dfun type). Run with full_output = 1 to get quantitative information.
warnings.warn(warning_msg, ODEintWarning)
/opt/anaconda3/lib/python3.7/site-packages/scipy/integrate/odepack.py:248: ODEintWarning: Excess work done on this call (perhaps wrong Dfun type). Run with full_output = 1 to get quantitative information.
warnings.warn(warning_msg, ODEintWarning)
/opt/anaconda3/lib/python3.7/site-packages/scipy/integrate/odepack.py:248: ODEintWarning: Excess work done on this call (perhaps wrong Dfun type). Run with full_output = 1 to get quantitative information.
warnings.warn(warning_msg, ODEintWarning)
/opt/anaconda3/lib/python3.7/site-packages/scipy/integrate/odepack.py:248: ODEintWarning: Excess work done on this call (perhaps wrong Dfun type). Run with full_output = 1 to get quantitative information.
warnings.warn(warning_msg, ODEintWarning)
/opt/anaconda3/lib/python3.7/site-packages/scipy/integrate/odepack.py:248: ODEintWarning: Excess work done on this call (perhaps wrong Dfun type). Run with full_output = 1 to get quantitative information.
warnings.warn(warning_msg, ODEintWarning)
/opt/anaconda3/lib/python3.7/site-packages/scipy/integrate/odepack.py:248: ODEintWarning: Excess work done on this call (perhaps wrong Dfun type). Run with full_output = 1 to get quantitative information.
warnings.warn(warning_msg, ODEintWarning)
[17]:
import pickle
with open("./China_II_mc_runs.pickle", "wb") as handle:
pickle.dump({"pars":saved_param, "pred":saved_prediction}, handle)
Análise do uso do sistema de saúde¶
Nesta análise, mostramos o erro percentual do quanto antes do pico, conseguimos prever a quantidade de pessoas que realmente serão identificadas como infectadas, uma vez que \(R(\infty)\) é a quantidade de pessoas recuperadas totais, daquelas que foram noficadas como infectadas no sistema de saúde. Desta forma segue o erro proporcional do erro a medida em que novos dados diários foram incluidos no modelo:
[34]:
x = range(start_day, len(I))
usage_error = [ 100 * abs(p*N - Rd[-1]) / Rd[-1] for p in saved_param['pop']]
fig9 = go.Figure()
fig9.add_trace(go.Scatter(
x=td[start_day:peak_pos],
y=usage_error[:peak_pos-start_day],
mode='lines',
name='Antes do pico',
line_shape='spline',
line = dict(color='royalblue', width=3),
hovertemplate="ε(%) = %{y:.0f}, <br> com %{x:.0f} dias de dados."))
fig9.add_trace(go.Scatter(
x=td[peak_pos:-1],
y=usage_error[peak_pos-start_day:-1],
mode='lines',
line_shape='spline',
name='Depois do pico',
line = dict(color='royalblue', width=3, dash='dot'),
hovertemplate="ε(%) = %{y:.0f}, <br> com %{x:.0f} dias de dados."))
fig9.add_trace(go.Scatter(
mode="markers", x=[peak_pos], y=[usage_error[peak_pos-start_day]],
marker_symbol="hexagram-dot", name="Momento do pico",
marker_line_color="midnightblue", marker_color="lightskyblue",
marker_line_width=2, marker_size=15,
hovertemplate="Pico no dia %{x}, com um ε(%) = %{y:.0f}."))
fig9.add_trace(go.Scatter(
mode="markers", x=[td[-1]], y=[usage_error[-1]],
marker_symbol="triangle-right-dot", name="Valor atual",
marker_line_color="#a00037", marker_color="#ff5c8d",
marker_line_width=2, marker_size=15,
hovertemplate="No dia %{x} da epidemia, <br> convergindo para ε(%) = %{y:.4f}."))
fig9.update_layout(template='xgridoff', yaxis_range=[-1,100],
legend_orientation="h", legend=dict(x=0.10, y=1.05),
xaxis_title='Dias (desde o começo da epidemia) utilizados para treinamento',
yaxis_title='Erro (%) do R(∞) - notificados',
title_text="Erro da previsão do uso do sistema de saúde - 🇨🇳 " + country['data']['name'])
fig9.show(renderer="png")
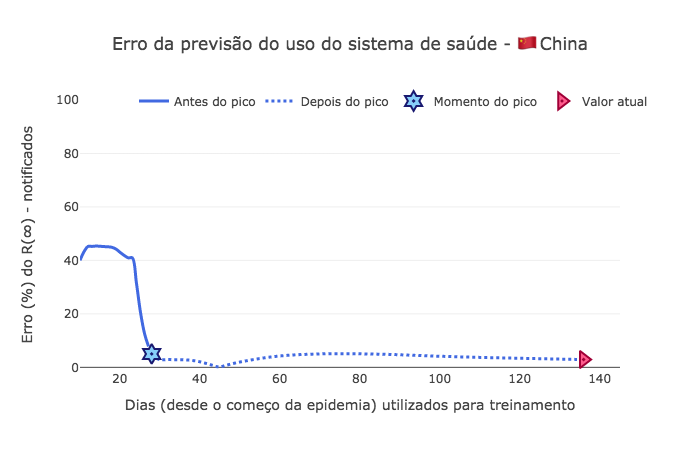
Visualizando as previsões de \(I(t)\)¶
Vamos analisar as previsões quando somente os dados antes do pico são fornecidos ao modelo, e as previões utilizando os dados após o pico:
[19]:
visual_peak = 30
p2 = figure(plot_height=500,
plot_width=600,
tools="",
toolbar_location=None,
title="Evolução do COVID - " + country['data']['name'])
# Preparando o estilo
p2.grid.grid_line_alpha = 0
p2.ygrid.band_fill_color = "olive"
p2.ygrid.band_fill_alpha = 0.1
p2.yaxis.axis_label = "Indivíduos"
p2.xaxis.axis_label = "Dias"
# Incluindo as curvas
p2.line(td, Id,
legend_label="Infectados",
line_cap="round", line_width=3, color="#c62828")
for data in saved_prediction[visual_peak-start_day:]:
p2.line(pred_t[:len(td)], -data[1][:len(td)],
legend_label="Previsão Infectados - Depois do pico",
line_cap="round", line_dash="dashed", line_width=4, color="#ffa000", line_alpha = 0.1)
for data in saved_prediction[:visual_peak-start_day]:
p2.line(pred_t[:len(td)], -data[1][:len(td)],
legend_label="Previsão Infectados - Antes do pico",
line_cap="round", line_dash="dashed", line_width=4, color="#42a5f5", line_alpha = 0.3)
# Colocando as legendas
p2.legend.click_policy="hide"
p2.legend.location = "top_right"
show(p2)
Visualizando os ajustes dos grupos¶
Aqui vamos analisar as previsões obtidas para cada grupo \(S(t)\), \(I(t)\) e \(R(t)\), a medida que mais dias foram informados ao modelo.
[26]:
p3 = figure(plot_height=350,
plot_width=600,
tools="",
toolbar_location=None,
title="Evolução do COVID - I(t) e R(t) - " + country['data']['name'])
p4 = figure(plot_height=350,
plot_width=600,
tools="",
toolbar_location=None,
title="Evolução do COVID - S(t) - " + country['data']['name'])
plot_all = True
# Preparando o estilo
p3.grid.grid_line_alpha = 0
p3.ygrid.band_fill_color = "olive"
p3.ygrid.band_fill_alpha = 0.1
p3.yaxis.axis_label = "Indivíduos"
p3.xaxis.axis_label = "Dias"
p4.grid.grid_line_alpha = 0
p4.ygrid.band_fill_color = "olive"
p4.ygrid.band_fill_alpha = 0.1
p4.yaxis.axis_label = "Indivíduos"
p4.xaxis.axis_label = "Dias"
# Incluindo as curvas
for data in saved_prediction[20:]:
p3.line(pred_t, data[1],
legend_label="Previsão Infectados",
line_cap="round", line_dash = 'dashed',
line_width=4, color="#42a5f5", line_alpha = 0.1)
p3.line(pred_t, data[2],
legend_label="Previsão Recuperados",
line_cap="round", line_dash = 'dashed', line_width=4,
color="#9c27b0", line_alpha = 0.07)
p3.line(td, Id,
legend_label="Infectados",
line_cap="round", line_width=3, color="#005cb2")
p3.line(td, Rd,
legend_label="Recuperados",
line_cap="round", line_width=3, color="#5e35b1")
if plot_all:
for data in saved_prediction:
p4.line(pred_t, data[0] + N*(1-saved_param['pop'][-1]),
legend_label="Previsão Suscetiveis",
line_cap="round", line_dash = 'dashed',
line_width=4, color="#ff5722", line_alpha = 0.07)
p4.line(td, Sd,
legend_label="Suscetiveis",
line_cap="round", line_width=3, color="#b71c1c")
# Colocando as legendas
p3.legend.click_policy="hide"
p3.legend.location = "top_left"
p4.legend.click_policy="hide"
p4.legend.location = "top_right"
show(column(p3,p4))
Análise de variação do \(R_0\)¶
[33]:
x = range(start_day, len(I))
beta_norm = [b*p for b,p in zip(saved_param['beta'],saved_param['pop'])]
Ro = [b*p/r for b,r,p in zip(saved_param['beta'],saved_param['r'],saved_param['pop'])]
fig1 = go.Figure()
fig1.add_trace(go.Scatter(
x=td[start_day:peak_pos],
y=beta_norm[:peak_pos-start_day],
mode='lines',
name='Antes do pico',
line_shape='spline',
line = dict(color='royalblue', width=3),
hovertemplate="β = %{y:.4f}, <br> com %{x:.0f} dias de dados."))
fig1.add_trace(go.Scatter(
x=td[peak_pos:-1],
y=beta_norm[peak_pos-start_day:-1],
mode='lines',
line_shape='spline',
name='Depois do pico',
line = dict(color='royalblue', width=3, dash='dot'),
hovertemplate="β = %{y:.4f}, <br> com %{x:.0f} dias de dados."))
fig1.add_trace(go.Scatter(
mode="markers", x=[peak_pos], y=[beta_norm[peak_pos-start_day]],
marker_symbol="hexagram-dot", name="Momento do pico",
marker_line_color="midnightblue", marker_color="lightskyblue",
marker_line_width=2, marker_size=15,
hovertemplate="Pico no dia %{x}, com um β = %{y:.4f}."))
fig1.add_trace(go.Scatter(
mode="markers", x=[td[-1]], y=[beta_norm[-1]],
marker_symbol="triangle-right-dot", name="Valor atual",
marker_line_color="#a00037", marker_color="#ff5c8d",
marker_line_width=2, marker_size=15,
hovertemplate="No dia %{x} da epidemia, <br> convergindo para β = %{y:.4f}."))
fig1.update_layout(template='xgridoff',
legend_orientation="h", legend=dict(x=0.10, y=1.05),
xaxis_title='Dias (desde o começo da epidemia) utilizados para treinamento',
yaxis_title='Parâmetro β',
title_text="Estimativa do parâmetro β - 🇨🇳 " + country['data']['name'])
fig1.show(renderer="png")
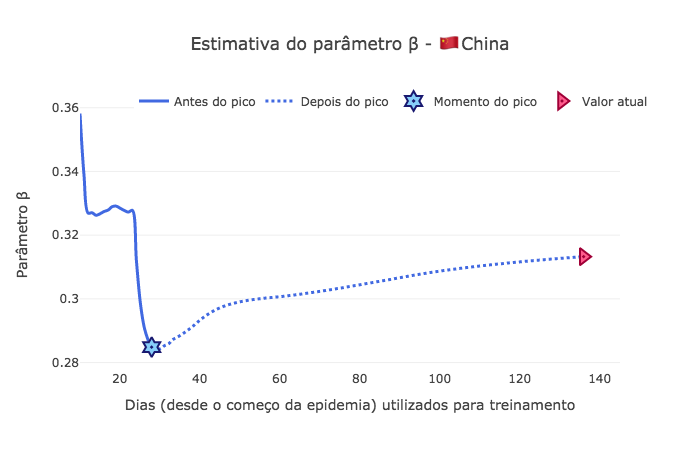
[32]:
fig2 = go.Figure()
fig2.add_trace(go.Scatter(
x=td[start_day:peak_pos],
y=saved_param['r'][:peak_pos-start_day],
mode='lines',
name='Antes do pico',
line_shape='spline',
line = dict(color='royalblue', width=3),
hovertemplate="r = %{y:.4f}, <br> com %{x:.0f} dias de dados."))
fig2.add_trace(go.Scatter(
x=td[peak_pos:-1],
y=saved_param['r'][peak_pos-start_day:-1],
mode='lines',
line_shape='spline',
name='Depois do pico',
line = dict(color='royalblue', width=3, dash='dot'),
hovertemplate="r = %{y:.4f}, <br> com %{x:.0f} dias de dados."))
fig2.add_trace(go.Scatter(
mode="markers", x=[peak_pos], y=[saved_param['r'][peak_pos-start_day]],
marker_symbol="hexagram-dot", name="Momento do pico",
marker_line_color="midnightblue", marker_color="lightskyblue",
marker_line_width=2, marker_size=15,
hovertemplate="Pico no dia %{x}, com um r = %{y:.4f}."))
fig2.add_trace(go.Scatter(
mode="markers", x=[td[-1]], y=[saved_param['r'][-1]],
marker_symbol="triangle-right-dot", name="Valor atual",
marker_line_color="#a00037", marker_color="#ff5c8d",
marker_line_width=2, marker_size=15,
hovertemplate="No dia %{x} da epidemia, <br> convergindo para r = %{y:.4f}."))
fig2.update_layout(template='xgridoff',
legend_orientation="h", legend=dict(x=0.07, y=1.06),
xaxis_title='Dias (desde o começo da epidemia) utilizados para treinamento',
yaxis_title='Parâmetro r',
title_text="Estimativa do parâmetro r - 🇨🇳 " + country['data']['name'])
fig2.show(renderer="png")
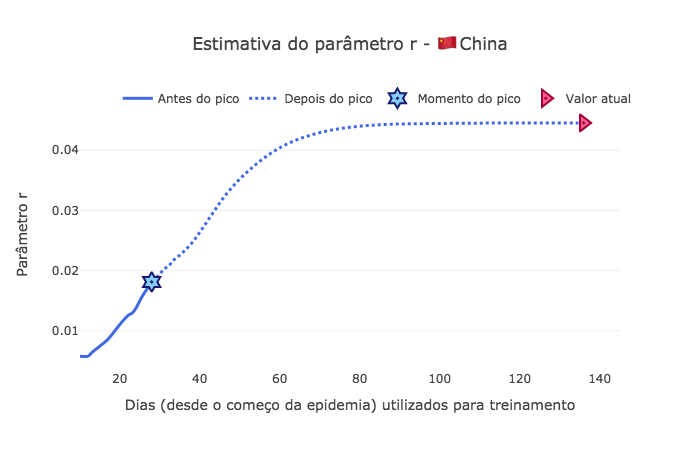
[31]:
fig3 = go.Figure()
fig3.add_trace(go.Scatter(
x=td[start_day:peak_pos],
y=Ro[:peak_pos-start_day],
mode='lines',
name='Antes do pico',
line_shape='spline',
line = dict(color='royalblue', width=3),
hovertemplate="r = %{y:.4f}, <br> com %{x:.0f} dias de dados."))
fig3.add_trace(go.Scatter(
x=td[peak_pos:-1],
y=Ro[peak_pos-start_day:-1],
mode='lines',
line_shape='spline',
name='Depois do pico',
line = dict(color='royalblue', width=3, dash='dot'),
hovertemplate="Ro = %{y:.4f}, <br> com %{x:.0f} dias de dados."))
fig3.add_trace(go.Scatter(
mode="markers", x=[peak_pos], y=[Ro[peak_pos-start_day]],
marker_symbol="hexagram-dot", name="Momento do pico",
marker_line_color="midnightblue", marker_color="lightskyblue",
marker_line_width=2, marker_size=15,
hovertemplate="Pico no dia %{x}, com um Ro = %{y:.4f}."))
fig3.add_trace(go.Scatter(
mode="markers", x=[td[-1]], y=[Ro[-1]],
marker_symbol="triangle-right-dot", name="Valor atual",
marker_line_color="#a00037", marker_color="#ff5c8d",
marker_line_width=2, marker_size=15,
hovertemplate="No dia %{x} da epidemia, <br> convergindo para Ro = %{y:.4f}."))
fig3.update_layout(template='xgridoff',
legend_orientation="h", legend=dict(x=0.07, y=1.06),
xaxis_title='Dias (desde o começo da epidemia) utilizados para treinamento',
yaxis_title='Parâmetro Ro',
title_text="Estimativa do parâmetro Ro - 🇨🇳 " + country['data']['name'])
fig3.show(renderer="png")
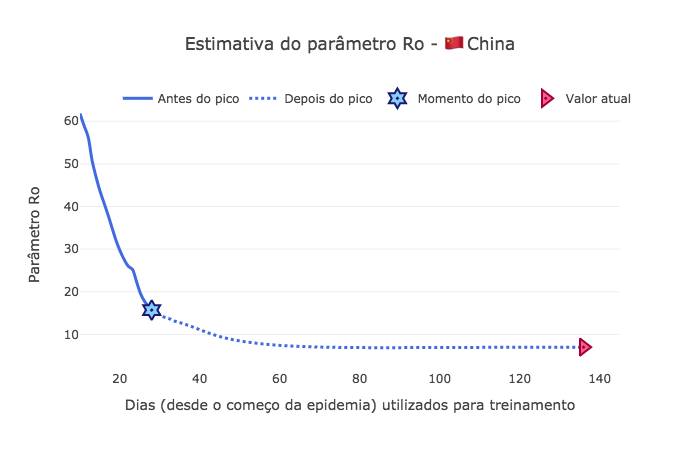
Análise de confiança¶
[20]:
from PyAstronomy import pyasl
dI = np.gradient(pyasl.smooth(I, 13, "hamming"))
t = np.linspace(0, len(dI), len(dI))
signal = np.array([di >= 0 for di in dI[::-1]])
import plotly.graph_objects as go
fig = go.Figure()
fig.add_trace(go.Scatter(
x=t[signal],
y=dI[::-1][signal],
mode='lines',
name='Antes do pico - Derivada positiva',
line_shape='spline',
line = dict(color='#512da8', width=3)))
fig.add_trace(go.Scatter(
x=t[~signal],
y=dI[::-1][~signal],
mode='lines',
line_shape='spline',
name='Depois do pico - Derivada negativa',
line = dict(color='#d8576b', width=3)))
fig.update_layout(template='xgridoff',
xaxis_title='Dias (invertido)',
yaxis_title='Taxa de variação de indivíduos',
title_text="Derivada da curva de Infectados - " + country['data']['name'])
# 'ggplot2', 'seaborn', 'simple_white', 'plotly',
# 'plotly_white', 'plotly_dark', 'presentation', 'xgridoff',
# 'ygridoff', 'gridon', 'none'
fig.show(renderer="png")
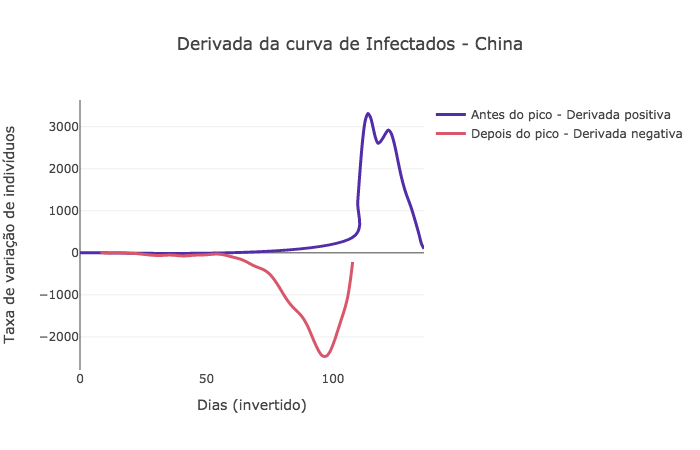
[22]:
peak_pos = np.argmax(~signal[::-1])
print("O pico da epidemia acontece no dia", peak_pos)
final_day = int(td[-1])
estimated_peaks = []
for data in saved_prediction:
dI = np.gradient(data[1][:final_day])
signal_pred = np.array([di >= 0 for di in dI[::-1]])
estimated_peaks.append(len(Id) - np.argmax(signal_pred))
estimated_peaks = np.array(estimated_peaks)
O pico da epidemia acontece no dia 28
[23]:
import plotly.graph_objects as go
peak_error = np.abs(estimated_peaks - peak_pos)
fig1 = go.Figure()
fig1.add_trace(go.Scatter(
x=td[start_day:peak_pos],
y=peak_error[:peak_pos-start_day],
mode='lines',
name='Antes do pico',
line_shape='spline',
line = dict(color='royalblue', width=3),
hovertemplate="Erro de %{y} dias, <br> com %{x:.0f} dias de dados."))
fig1.add_trace(go.Scatter(
x=td[peak_pos:],
y=peak_error[peak_pos-start_day:],
mode='lines',
line_shape='spline',
name='Depois do pico',
line = dict(color='royalblue', width=3, dash='dot'),
hovertemplate="Erro de %{y} dias, <br> com %{x:.0f} dias de dados."))
fig1.add_trace(go.Scatter(
mode="markers", x=[peak_pos], y=[peak_error[peak_pos-start_day]],
marker_symbol="hexagram-dot", name="Momento do pico",
marker_line_color="midnightblue", marker_color="lightskyblue",
marker_line_width=2, marker_size=15,
hovertemplate="Pico no dia %{x} depois do começo da epidemia."))
fig1.update_layout(yaxis_range=[-1,31],
template='xgridoff',
legend_orientation="h", legend=dict(x=0.20, y=1.0),
xaxis_title='Dias (desde o começo da epidemia) utilizados para treinamento',
yaxis_title='Erro (em dias) da estimativa do pico',
title_text="Erro da estimativa do pico - " + country['data']['name'])
# 'ggplot2', 'seaborn', 'simple_white', 'plotly',
# 'plotly_white', 'plotly_dark', 'presentation', 'xgridoff',
# 'ygridoff', 'gridon', 'none'
fig1.show(renderer="png")
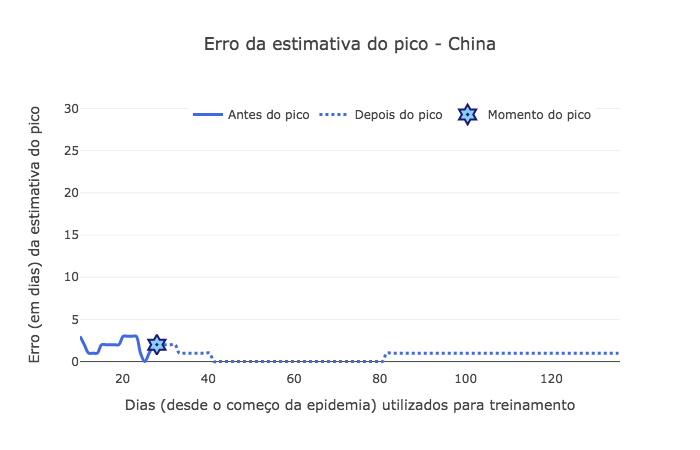